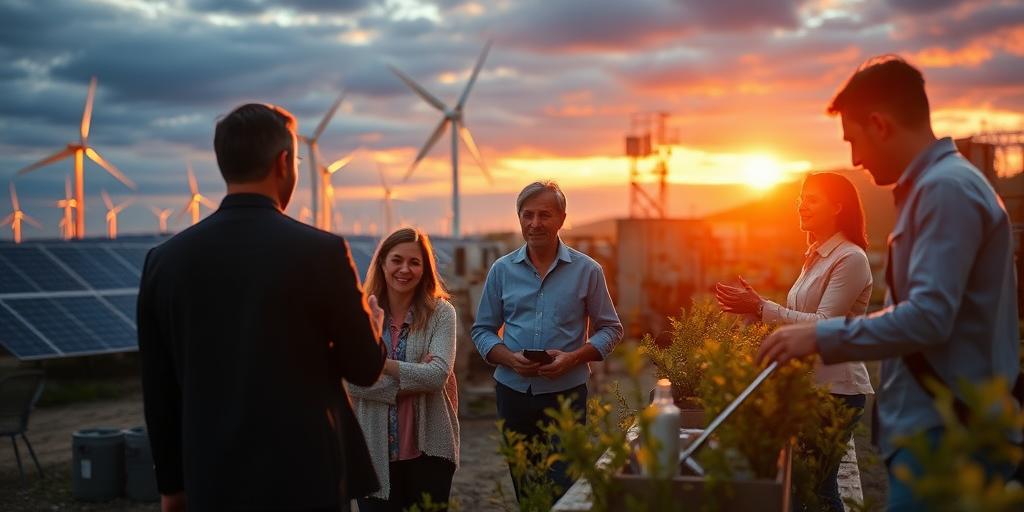
AI Renewable Energy Optimization: Harnessing Intelligence for a Sustainable Future
Discover how AI renewable energy optimization is transforming the energy landscape, enhancing efficiency, reducing waste, and creating a sustainable future. Explore innovative solutions, case studies, and expert insights.
Introduction
Did you know that by 2025, it’s projected that around 50% of global energy will come from renewable sources? As the world increasingly turns towards sustainable energy, integrating artificial intelligence (AI) into this transition is becoming crucial. AI renewable energy optimization combines cutting-edge technology with eco-friendly practices, driving efficiencies and reducing costs across the board! In this article, we’ll explore how AI is revolutionizing energy optimization, share success stories, and discuss the future of green energy. Are you ready to unpack the power of AI in renewable energy?
Understanding AI in Renewable Energy
Artificial Intelligence (AI) has rapidly become a transformative force in numerous sectors, and renewable energy is no exception. At its core, AI refers to the simulation of human intelligence processes by machines, especially computer systems. In the renewable energy sector, AI’s applications are manifold, encompassing everything from predictive analytics to automated energy management systems.
When we delve into AI’s role in renewable energy, several technologies come into play. Machine learning, a subset of AI, allows systems to learn from data, improving their performance over time without being explicitly programmed. Data analytics provides the tools necessary to interpret vast datasets generated from renewable energy sources, enabling better insights and decision-making. Together, these technologies enhance the operational capabilities of renewable energy systems, leading to improved efficiency and reliability.
One of the most significant contributions of AI in this sector is its ability to improve predictions and decision-making. By analyzing historical data and identifying patterns, AI algorithms can forecast energy production, predict maintenance needs, and optimize energy distribution. This predictive capability not only enhances operational efficiency but also plays a crucial role in integrating renewable energy sources into existing energy grids.
Benefits of AI Optimization in Renewable Energy Systems
The impact of AI on renewable energy systems is profound, with several key benefits emerging from its application.
One of the primary advantages is enhanced efficiency. By leveraging AI to predict energy production accurately, operators can adjust their strategies to maximize output. For example, AI can analyze weather patterns and historical data from solar panels to predict energy generation for the upcoming days. This allows for better planning and integration with energy consumption patterns, reducing reliance on fossil fuels during peak demand periods.
Moreover, AI contributes significantly to reducing operational costs. Through real-time data analysis, AI systems can identify inefficiencies and suggest corrective measures. For instance, energy providers can use AI algorithms to monitor the performance of wind turbines, determining when they are underperforming or requiring maintenance. This proactive approach reduces downtime and extends the lifespan of equipment, resulting in significant cost savings.
Additionally, AI helps mitigate waste and optimize energy storage solutions. As renewable energy sources like solar and wind are inherently variable, AI can optimize the use of energy storage systems, ensuring that electricity generated is used efficiently. By analyzing demand forecasts and energy generation patterns, AI can determine the optimal times to store energy and when to release it back into the grid. This capability is critical in balancing supply and demand, ultimately leading to a more stable energy system.
Case Studies: Successful Implementation of AI in Renewable Energy
Several notable projects illustrate the successful application of AI in optimizing renewable energy.
One prominent case is the implementation of AI in solar energy management at a large solar farm in California. By utilizing machine learning algorithms, the facility improved its energy output by 20% over a year. The AI system analyzed historical weather data, panel performance, and energy demand patterns to optimize the energy generation schedule throughout the day. The results were impressive, leading to increased efficiency and substantial financial savings.
In the wind energy sector, a European wind farm operator deployed AI to enhance turbine performance. The operator used predictive maintenance algorithms to analyze vibration data and weather conditions, allowing them to anticipate failures before they occurred. This approach not only reduced maintenance costs but also minimized downtime, ultimately increasing energy production by 15% annually.
Hydropower is another area where AI has made significant strides. A case study from a hydroelectric facility in Canada demonstrated how AI could optimize water flow and turbine performance based on real-time data analytics. By adjusting water release strategies according to electricity demand and weather conditions, the facility improved its energy output by 12% while also adhering to environmental regulations.
These case studies highlight not only the efficiency gains and cost savings achieved through AI but also the broader lessons learned regarding the integration of advanced technologies into traditional energy systems. The ability to adapt and evolve is crucial as the renewable energy landscape continues to shift.
AI Tools and Technologies Revolutionizing Renewable Energy
The integration of AI into renewable energy systems is powered by a range of specific tools and software designed for energy optimization.
Leading the charge are advanced machine learning platforms that analyze data from renewable energy sources. These platforms can process vast amounts of information, including weather forecasts, grid demand, and equipment performance metrics. Tools like TensorFlow and PyTorch are commonly used to build predictive models that can forecast energy production and optimize operations.
The Internet of Things (IoT) also plays a critical role in data collection and real-time monitoring. Smart sensors installed in solar panels and wind turbines gather essential data that feeds into AI systems. These devices can track performance metrics such as temperature, energy output, and operational status, sending real-time information to centralized AI platforms for analysis. This connectivity allows for better decision-making and immediate responses to changing conditions.
Smart grids represent another technological advancement revolutionizing renewable energy. By integrating AI and IoT, smart grids can manage electricity flow more effectively, ensuring that renewable energy sources are utilized optimally. Predictive analytics within smart grids enable energy providers to forecast demand accurately, facilitating a smoother interface between generation and consumption. This synchronization is essential in maximizing the potential of renewable energy sources.
Challenges in Implementing AI for Energy Optimization
Despite the clear benefits of integrating AI into renewable energy systems, several challenges remain that must be addressed for broader implementation.
One significant barrier is the high initial costs associated with adopting AI technologies. Small and medium-sized energy producers may find it challenging to invest in the necessary infrastructure and talent required to implement AI solutions effectively. Without financial backing or incentives, many may hesitate to take the plunge into AI adoption.
Another pressing concern is the expertise gap within the industry. While AI technology has advanced rapidly, there is a shortage of professionals skilled in both renewable energy and AI. This skills gap can hinder the effective deployment of AI systems, as organizations struggle to find qualified personnel who understand the intricacies of both fields.
Data privacy and cybersecurity are also critical issues that need to be addressed. As AI systems rely heavily on data collection and analysis, concerns about how data is managed and safeguarded are paramount. Energy providers must implement robust security measures to protect sensitive information from cyber threats, ensuring that both operational integrity and consumer trust are maintained.
Finally, the need for regulatory frameworks and standardization cannot be overlooked. As AI technologies evolve, regulatory bodies must adapt to ensure that the integration of AI aligns with existing laws and regulations. Establishing clear guidelines will help mitigate risks and foster an environment where AI can thrive in the renewable energy sector.
The Future of AI in Renewable Energy Optimization
Looking ahead, the future of AI in renewable energy optimization is filled with promise. We can predict several trends and innovations that will shape the industry in the coming years.
One anticipated trend is the continued advancement of machine learning algorithms that become increasingly sophisticated in their predictive capabilities. As more data is generated from renewable energy systems, AI will be able to provide even more accurate forecasts for energy production and demand. This evolution will enhance the integration of renewable sources into the grid, leading to a more resilient energy infrastructure.
Furthermore, the role of collaborations between technology companies and energy providers will become increasingly vital. By partnering, these entities can combine their expertise to develop more effective solutions and share resources, ultimately accelerating the adoption of AI technologies in renewable energy. Joint ventures and partnerships can lead to innovative projects that drive efficiency and sustainability.
The impact of AI on global energy policy and infrastructure is another area to watch. As countries strive to meet their renewable energy targets, AI will likely play a crucial role in shaping policies that promote sustainability. Governments may introduce incentives for the adoption of AI technologies, recognizing their potential to enhance energy efficiency and reduce carbon emissions.
In summary, the integration of AI into renewable energy systems presents an exciting opportunity to revolutionize the sector. While challenges remain, the benefits of improved efficiency, reduced costs, and optimized operations make a compelling case for the continued investment in AI technologies. The collaboration between tech companies, energy providers, and regulatory bodies will be instrumental in realizing the full potential of AI in renewable energy.
Conclusion
AI renewable energy optimization is not just a trend; it’s the future of sustainable energy. Harnessing the power of AI amplifies the potential of renewable sources, leading us towards a greener environment and a more efficient energy grid. As we navigate challenges and embrace innovations, the collaboration between technology and sustainability will yield promising results. Ready to explore where AI can take us next in the renewable energy space?