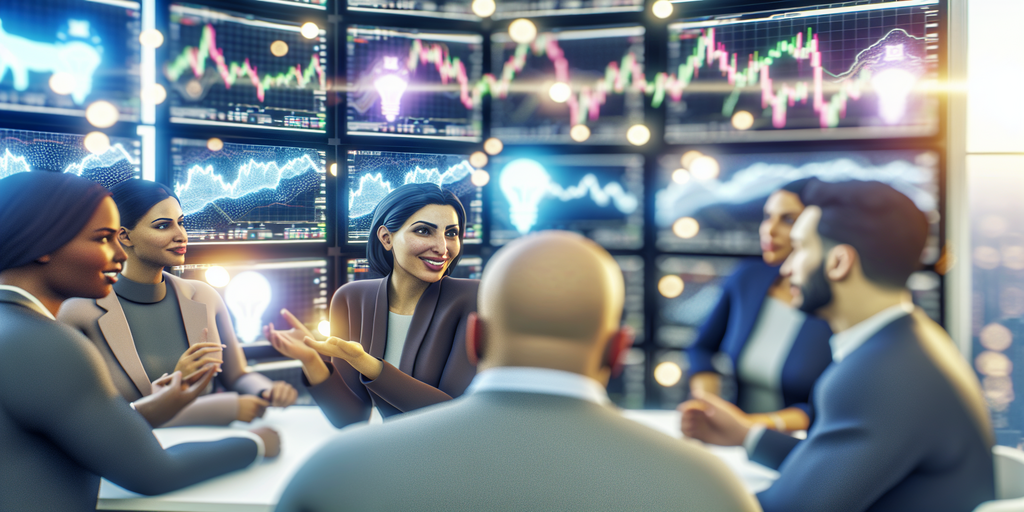
AI Stock Prediction: Revolutionizing Financial Forecasting in 2025
Discover how AI stock prediction is transforming investment strategies. Learn about machine learning models, algorithms, data analysis, and more in this comprehensive guide.
Introduction
Did you know that by 2025, estimates suggest that machine learning will enhance stock market predictions by up to 80%? Imagine having access to cutting-edge technology that can analyze vast amounts of data and uncover trends that human analysts might miss! Welcome to the world of AI stock prediction, where innovative algorithms and sophisticated models are redefining how we approach investment strategies. In this article, weâll explore the different facets of AI in stock prediction, from the underlying technology to practical applications, offering insights that are both valuable and engaging for seasoned investors and novices alike!
The Fundamentals of AI in Stock Prediction
Artificial Intelligence (AI) has emerged as a game-changer in the world of investments, particularly in stock prediction. The significance of AI lies in its ability to analyze vast amounts of data quickly and accurately, enabling investors to make informed decisions. Key technologies driving this transformation include machine learning, neural networks, and data mining, each contributing uniquely to the predictive capabilities of AI.
The evolution of stock prediction techniques has been remarkable. Traditional methods relied heavily on fundamental analysis, technical indicators, and human intuition. However, with the advent of AI-driven models, the landscape has shifted toward more sophisticated, data-driven approaches. These models leverage complex algorithms to identify patterns and trends that were previously difficult to detect.
How Machine Learning Models Work for Stock Forecasting
Understanding how machine learning models function is crucial in grasping their application in stock forecasting. There are two main categories of machine learning: supervised and unsupervised learning. Supervised learning involves training a model on historical data with known outcomes, while unsupervised learning seeks to identify patterns in data without prior labels. In stock prediction, supervised learning is commonly used to forecast future prices based on historical trends.
Popular algorithms that underpin stock forecasting include decision trees and regression analysis. Decision trees provide a visual representation of decisions and their possible consequences, while regression analysis helps in understanding relationships between variables. Both are essential for making accurate predictions.
A critical aspect of creating reliable models is the importance of training and testing datasets. A well-structured training dataset allows the model to learn, while a testing dataset evaluates its performance. Balancing these datasets is vital for ensuring that the model generalizes well to unseen data.
Data Sources and Analysis Techniques
When it comes to stock prediction, the type of data used plays a significant role. Analysts commonly rely on historical prices, economic indicators, and even social media sentiment. Each of these data sources provides unique insights that, when combined, can enhance prediction accuracy.
Data preprocessing is a crucial step in the analysis process. Techniques such as normalization, cleaning, and feature selection help to prepare the data for analysis. Normalization adjusts the data to a common scale, cleaning removes inaccuracies, and feature selection identifies the most relevant variables for prediction.
Big data analytics further enhances the power of AI in stock prediction. By processing large datasets quickly, big data tools can uncover hidden patterns and correlations that would otherwise go unnoticed, ultimately improving prediction accuracy.
Potential Benefits and Risks of AI Stock Prediction
The integration of AI in stock forecasting presents several advantages. Speed and accuracy are two primary benefits; AI can analyze vast datasets in real-time, enabling timely decision-making. Additionally, AIâs ability to process and interpret complex data gives it an edge over traditional investment strategies.
However, there are also limitations and challenges to consider. Issues such as overfitting, where models perform well on training data but poorly on new data, and biases in the algorithms can lead to inaccurate predictions. Furthermore, market volatility can drastically affect the reliability of predictions, making it essential for investors to approach AI-generated forecasts with caution.
Ethical considerations also come into play when using AI for financial predictions. The potential for biased algorithms and the implications of high-frequency trading raise important questions about fairness and transparency in the market.
Real-World Applications of AI in Stock Trading
Many investment firms have successfully adopted AI-driven approaches to enhance their trading strategies. Case studies of these firms highlight the effectiveness of AI in identifying lucrative investment opportunities and managing risks.
Various AI tools and platforms are now available for traders and analysts. These tools range from algorithmic trading platforms to sentiment analysis software, providing users with the ability to leverage AI insights in their decision-making processes.
Retail investors can also benefit from AI predictions. By utilizing available AI-driven tools, they can gain access to sophisticated analyses and insights that were once exclusive to institutional investors.
Future Trends in AI Stock Prediction
As we look to the future, emerging technologies are poised to shape the landscape of stock forecasting. Quantum computing, for instance, has the potential to process information at unprecedented speeds, opening new avenues for AI applications in finance.
Predictions suggest that AI will continue to evolve in the coming decade, integrating more advanced algorithms and becoming increasingly adept at adapting to market changes. However, the role of regulatory frameworks will be crucial in guiding the responsible adoption of AI in finance, ensuring that innovation aligns with ethical standards and market integrity.
Conclusion
AI stock prediction is not just a fleeting trend; itâs a paradigm shift in the investment landscape! By understanding the fundamentals, models, and potential of AI technologies, investors can make informed decisions that align with future market dynamics. Donât be left behindâexplore the capabilities of AI in your investment strategy today and stay ahead of the curve! Ready to take the plunge into the world of AI-enhanced trading?