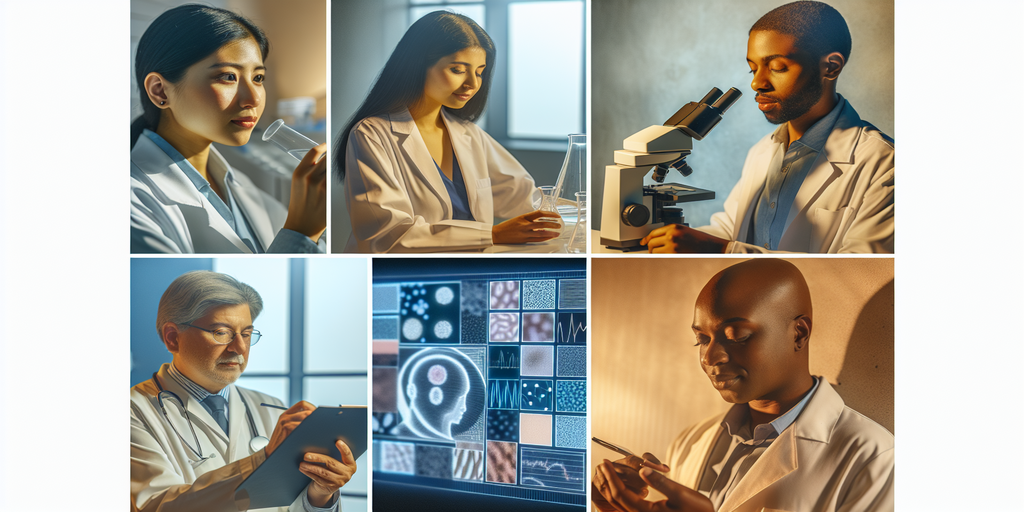
Unleashing the Future: AI in Precision Medicine Drug Discovery
Discover how AI is revolutionizing precision medicine drug discovery by enhancing accuracy, speed, and efficiency. Explore key technologies, trends, and ethical considerations in this transformative field!
Introduction
Did you know that 95% of drugs fail during the clinical trial phase? This staggering statistic highlights the urgent need for innovation in drug discovery. Enter artificial intelligence (AI) – a game-changer that’s reshaping the landscape of precision medicine! In this article, we will explore how AI is enhancing the accuracy and efficiency of drug discovery, paving the way for more personalized and effective treatments. From machine learning algorithms to genomic data analysis, we’ll delve into the fascinating technologies driving this revolution in healthcare.
Understanding AI in Precision Medicine
When we think about the intersection of artificial intelligence (AI) and precision medicine, it’s essential to start with a clear definition of both concepts. AI refers to the simulation of human intelligence processes by computer systems, which include learning, reasoning, and self-correction. On the other hand, precision medicine is a medical model that proposes the customization of healthcare, with decisions and treatments tailored to individual patients based on their genetic, environmental, and lifestyle factors.
At its core, the integration of AI into precision medicine revolves around analyzing massive datasets to understand how genetic variations affect drug responses. This means AI isn’t just an ancillary tool; it’s becoming a pivotal part of how we approach patient care, especially when it comes to personalizing treatments.
Components of AI in Precision Medicine
AI’s role in precision medicine can be broken down into several critical components: machine learning, data analytics, and computational biology.
Machine learning is a subset of AI that allows systems to learn from data and improve their performance over time without being explicitly programmed. In the context of drug discovery, machine learning algorithms can identify patterns in complex datasets that might be invisible to human researchers.
Data analytics complements machine learning by providing the tools and techniques to interpret vast amounts of data generated in healthcare settings. This includes everything from electronic health records to clinical trial results, enabling researchers to derive actionable insights.
Lastly, computational biology involves the application of computational techniques to understand biological data. This field plays a crucial role in drug discovery, providing the computational power needed to simulate biological processes, analyze genomic sequences, and model interactions between drugs and biological systems.
Importance of Integrating AI into Drug Discovery
The integration of AI into drug discovery represents a transformative moment for healthcare. One of the primary reasons for this integration is the sheer volume of data generated in the biomedical field. Traditional methods of drug discovery are often too slow and inefficient to keep up with the rapidly growing body of research.
By incorporating AI, researchers can process and analyze data at unprecedented speeds. This not only accelerates the discovery of new drugs but also enhances the ability to predict their effectiveness and safety. Moreover, the traditional trial-and-error approach to drug development is being revolutionized by AI’s predictive capabilities, which can significantly reduce the time and resources required to bring a drug to market.
Key Technologies Transforming Drug Discovery
Within the realm of AI, machine learning algorithms are at the forefront of transforming drug discovery. There are several commonly used algorithms that researchers employ, including decision trees, support vector machines, and neural networks.
Decision trees help in classifying different biological entities and predicting outcomes based on various input features. Support vector machines are particularly useful in high-dimensional spaces, making them ideal for genomic data classification. Meanwhile, neural networks, especially deep learning architectures, are adept at handling complex datasets and can uncover intricate patterns related to drug interactions and patient responses.
The ability to analyze genomic data is one of the most significant advantages of AI in precision medicine. With the explosion of genomic sequencing technologies, the amount of genomic data generated is staggering. AI algorithms can sift through these vast datasets, identifying critical mutations and variations that could guide therapeutic decisions.
Natural Language Processing (NLP) is another vital technology in the AI toolbox for drug discovery. One of the most time-consuming aspects of research is reviewing vast amounts of literature and extracting relevant data. NLP algorithms can expedite this process by automatically scanning and summarizing research papers, clinical trial results, and other documents.
Benefits of AI in Drug Development
The advantages of integrating AI into drug development processes are profound and multifaceted.
One of the most significant benefits is the speed at which drug discovery timelines can be reduced. Traditional drug development can take 10-15 years and cost billions of dollars. AI-driven approaches can cut down this timeline dramatically, enabling faster identification of viable drug candidates.
In addition to speed, AI can contribute to a more cost-effective drug development process. By reducing the time and resources required for research and clinical trials, pharmaceutical companies can lower their overall expenditure.
Accuracy in predicting drug efficacy and safety profiles is another critical benefit of AI integration. Traditional methods may rely on limited datasets or historical data that do not fully represent the current landscape.
Challenges and Ethical Considerations
Despite its potential, the integration of AI into drug discovery is not without challenges and ethical considerations.
One of the foremost concerns is data privacy. The use of sensitive patient data in AI models raises significant questions about security and compliance with regulations like the Health Insurance Portability and Accountability Act (HIPAA).
Another critical challenge is the potential for bias in AI algorithms. If the data used to train these models is not representative of the broader population, the resulting models may yield biased outcomes.
As AI continues to permeate the healthcare sector, there is a pressing need for new regulatory frameworks to govern its use. Current regulations may not adequately address the unique challenges posed by AI technologies.
Future Trends in AI-Driven Drug Discovery
As we look ahead, several trends are emerging in the field of AI-driven drug discovery that promise to shape the future of healthcare.
One of the most exciting developments is the move toward personalized medicine. AI is enabling the creation of tailored therapies that take into account an individual’s genetic profile, lifestyle, and health history.
We are also witnessing the rise of collaborative platforms that unite AI startups with pharmaceutical companies. These partnerships leverage the strengths of both sectors—startups bring innovative AI technologies, while established companies provide the resources and clinical expertise necessary to bring new drugs to market.
Finally, the utilization of real-world evidence is becoming increasingly important. AI can analyze data from various sources, including electronic health records and post-market data, to gain insights into how drugs perform in real-world settings.
As we navigate the complexities of integrating AI into drug discovery and precision medicine, it’s clear that the journey is just beginning. The potential benefits are immense, but they come with significant responsibilities to ensure ethical and equitable practices. The future of healthcare will undoubtedly be shaped by the innovations and challenges that lie ahead in this dynamic field.
Conclusion
As we stand on the brink of a transformative era in healthcare, the integration of AI in precision medicine drug discovery promises to enhance the effectiveness and efficiency of pharmaceutical development. By embracing AI technologies, the healthcare industry can overcome daunting challenges and unlock new possibilities for personalized treatment. Stay informed and consider how these advancements may affect patient care and your future health decisions. The revolution is here – are you ready to embrace it?