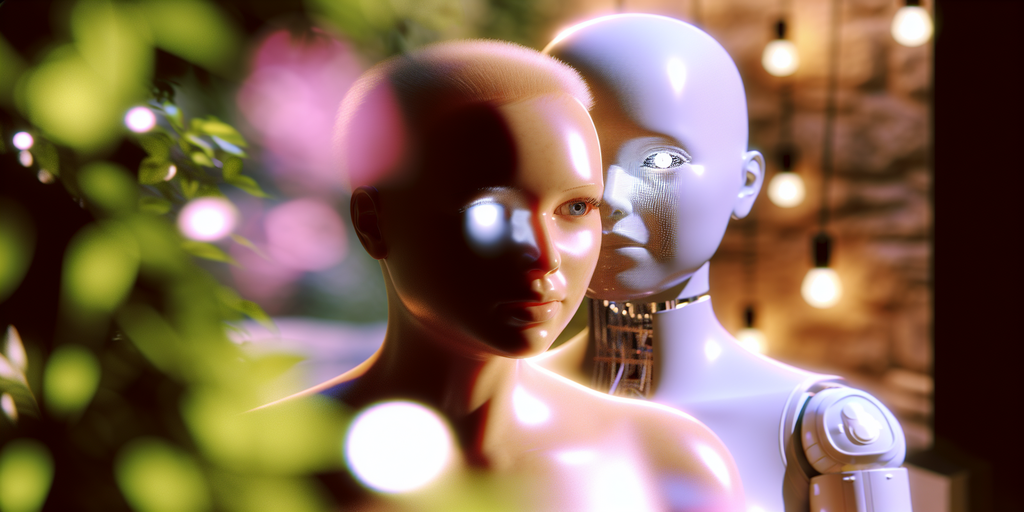
Unlocking AI Security: Strategies to Safeguard Your Digital Future
Discover the world of AI security! Learn about effective strategies and best practices to protect your systems from emerging threats and vulnerabilities in the realm of artificial intelligence.
Introduction
Did you know that 85% of organizations report an increase in AI-related security concerns? As we embrace the transformative power of artificial intelligence, we must also contend with new vulnerabilities that can jeopardize our data and systems. AI security is no longer a luxury—it’s a necessity! This article delves into the crucial strategies and best practices to safeguard our digital landscape from the challenges posed by AI technologies. Join me on this journey to unravel the complexities of AI security and to arm yourself with knowledge that secures both your organization and its future.
Understanding AI Security Threats and Vulnerabilities
When we talk about AI security, we’re diving into a realm that encompasses not just the technology itself but also the data it uses and the infrastructure that supports it. AI security refers to the measures taken to protect AI systems from threats, vulnerabilities, and attacks. This includes safeguarding data, algorithms, and the processes that govern AI operations.
One of the primary concerns in AI security is the common vulnerabilities that can be found in these systems. For instance, adversarial attacks can manipulate AI models by subtly altering the input data, leading to incorrect outputs. Additionally, data poisoning attacks can compromise the training data, which in turn affects the AI’s learning process. These vulnerabilities can have serious implications, ranging from skewed results to complete system failures.
Real-world examples illustrate these vulnerabilities vividly. For instance, there have been incidents where AI algorithms used in facial recognition systems were fooled by simple alterations to images. Such breaches showcase not only the fragility of AI systems but also highlight the urgent need for robust security measures.
Essential Strategies for Enhancing AI Security
To bolster AI security, implementing robust security frameworks is essential. Organizations should adopt comprehensive security protocols that encompass all aspects of AI development and deployment. This includes risk assessments, threat modeling, and establishing clear guidelines for data usage.
Another critical aspect is the importance of privacy protocols. With AI systems often handling sensitive data, ensuring compliance with privacy regulations is paramount. This involves anonymizing data, enforcing strict access controls, and integrating privacy-by-design principles into the development process.
Regular updates and patches for software are vital in maintaining AI security. Just like any other software, AI systems are susceptible to vulnerabilities that can be exploited. By keeping software up to date, organizations can protect themselves from potential breaches and ensure that their systems remain resilient against evolving threats.
Best Practices for Secure AI Development
For AI developers, secure coding practices are fundamental. This involves writing code that is not only functional but also resilient to attacks. Adopting secure coding standards, conducting code reviews, and utilizing automated security testing tools can significantly reduce vulnerabilities in AI applications.
Moreover, the role of continuous monitoring and auditing cannot be overstated. Organizations should implement systems that constantly monitor AI performance and security. Regular audits can help identify potential weaknesses before they are exploited, ensuring that the AI systems remain secure over time.
Equally important is building a culture of security within AI teams. This means fostering an environment where security is a shared responsibility. Training team members on security best practices and encouraging open dialogue about potential threats can create a proactive approach to AI security.
Utilizing Emerging Technologies to Boost AI Security
As the field of AI security evolves, emerging technologies are playing a crucial role in enhancing protection measures. For example, blockchain technology brings a decentralized approach to data security, making it more difficult for hackers to alter or steal sensitive information. By integrating blockchain with AI, organizations can create more secure data storage solutions.
Machine learning also has a significant role in threat detection. By analyzing patterns and anomalies within data, machine learning algorithms can identify potential breaches in real time, allowing organizations to respond swiftly to emerging threats.
Additionally, the evolution of encryption methods in AI is vital for protecting sensitive data. Advanced encryption techniques can safeguard data at rest and in transit, ensuring that unauthorized access is prevented even if data is intercepted.
Regulatory Compliance and Ethical Considerations
Navigating the landscape of AI security requires an understanding of current regulations impacting the field. Compliance with regulations such as GDPR, CCPA, and others is essential for protecting user data and maintaining trust. Organizations must stay informed about these regulations and adapt their practices accordingly.
Equally important is the emphasis on ethical AI practices. Developers and organizations must consider the ethical implications of their AI systems. This involves ensuring that AI systems are fair, transparent, and accountable in their decision-making processes.
Balancing innovation with security obligations is a delicate act. While organizations strive to push the boundaries of technology and deliver advanced AI solutions, they must not compromise on security. Finding this balance helps ensure that AI developments are both progressive and secure.
Case Studies: Successful AI Security Implementations
Looking at real-world success stories can provide valuable insights into effective AI security measures. Many businesses have excelled in implementing robust AI security strategies. For instance, a leading financial institution invested heavily in AI-driven threat detection systems that significantly reduced fraud incidents.
Lessons learned from these successful implementations often highlight the importance of proactive security measures and adaptive strategies. Organizations that continuously refine their security practices tend to be more resilient against emerging threats.
Innovative solutions to complex AI security challenges also emerge from these case studies. For example, companies are increasingly utilizing collaborative filtering techniques and federated learning to enhance data privacy while still benefiting from AI’s analytical capabilities. These strategies demonstrate that security and innovation can go hand in hand in the ever-evolving landscape of AI.
Conclusion
As we navigate the rapidly evolving landscape of AI, understanding and implementing effective security measures is paramount. From identifying vulnerabilities to employing cutting-edge technologies, every step we take is crucial to fortifying our defenses. Embrace these strategies within your organization and lead the charge towards a secure AI future! Don’t wait—start integrating these security measures today to safeguard your digital assets!