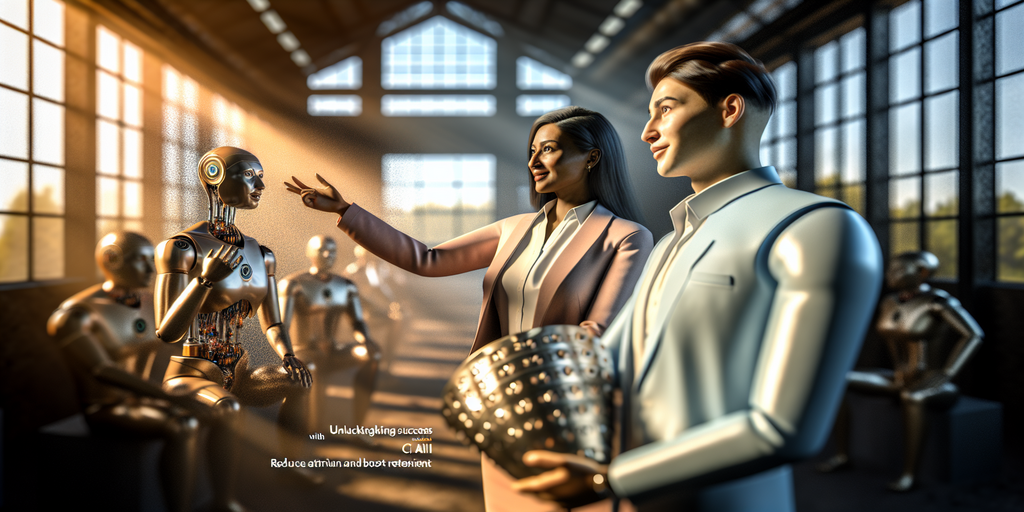
Unlocking Success with AI Predictive Customer Churn Analysis: Reduce Attrition and Boost Retention in 2025
Discover how AI predictive customer churn analysis can transform your business. Learn the strategies to identify at-risk customers and improve retention rates effectively!
Introduction
In today’s competitive market, understanding customer behavior is more crucial than ever! Did you know that acquiring a new customer can cost five times more than retaining an existing one? That’s right— and this is where AI predictive customer churn analysis comes in to save the day! By leveraging artificial intelligence to analyze customer data, businesses can predict which customers are likely to leave and take proactive measures to retain them. This article will guide you through the essentials of AI-driven churn analysis, its importance, and actionable strategies you can implement to enhance your customer retention efforts. Get ready to dive into the future of customer loyalty!
What is Customer Churn and Why Does it Matter?
Customer churn refers to the rate at which customers stop doing business with a company. It can be broken down into two types: voluntary and involuntary. Voluntary churn occurs when a customer chooses to leave, often due to dissatisfaction with the product or service, while involuntary churn happens when customers leave due to factors outside their control, such as financial difficulties or changes in circumstances.
Understanding churn is vital for business growth. High churn rates can signify deeper issues within a company, such as poor customer service or lack of engagement. Companies must recognize that retaining existing customers is typically less costly than acquiring new ones. Therefore, a comprehensive understanding of churn dynamics can help businesses strategize effectively, improve customer experiences, and ultimately enhance their bottom line.
High churn rates can have a significant impact on revenue and brand reputation. When customers leave, not only does it decrease immediate revenue, but it can also affect the company’s image in the marketplace. Customers often share their experiences through word-of-mouth and online reviews, which can deter potential customers from engaging with the brand.
The Role of AI in Predictive Customer Churn Analysis
AI technologies, particularly machine learning and natural language processing (NLP), are increasingly being used for churn analysis. Machine learning allows companies to analyze patterns in customer behavior data, while NLP can help interpret customer feedback and sentiment from various sources, such as social media and surveys.
AI processes vast amounts of customer data quickly and efficiently, enabling businesses to identify trends and predict churn more accurately. This capability is a game-changer compared to traditional methods of churn prediction, which can be time-consuming and less precise. By leveraging AI, companies can gain actionable insights that allow them to address potential churn proactively rather than reactively.
Key Metrics for Measuring Customer Churn
To effectively measure customer churn, it is essential to define the Customer Churn Rate and understand how to calculate it. The churn rate is typically calculated by taking the number of customers lost during a specific period and dividing it by the total number of customers at the beginning of that period.
In addition to the churn rate, other relevant metrics include Customer Lifetime Value (CLV) and Net Promoter Score (NPS). CLV helps businesses understand the long-term revenue potential of a customer, while NPS measures customer loyalty and satisfaction. Selecting the right metrics for analysis is crucial as they provide a holistic view of customer relationships and help pinpoint areas needing improvement.
Steps to Implement AI Predictive Customer Churn Analysis
Implementing AI predictive customer churn analysis involves several key steps. First, gather and prepare customer data for analysis, ensuring that it is clean and structured. This data could range from transaction history to customer interactions.
Next, build and train predictive models using various machine learning algorithms. This involves feeding the prepared data into the model so it can learn and make predictions based on patterns identified in the data.
Finally, it’s important to test, validate, and iterate on the models to improve their accuracy over time. Continuous refinement of the predictive models enables businesses to adapt to changing customer behaviors and improve their retention strategies.
Tools and Technologies for Churn Prediction
Several popular AI tools and software can aid in churn analysis. Tools like Salesforce and IBM Watson are widely recognized for their capabilities in data analysis and customer relationship management.
Integration with existing CRM systems is an essential consideration, as it allows for smoother data flows and more cohesive insights. Additionally, many of these tools are cost-effective and user-friendly, making them accessible for businesses of all sizes. Choosing the right tools can greatly enhance the efficiency of churn prediction efforts.
Developing Effective Customer Retention Strategies
Once churn predictions are made, businesses can identify actionable insights that emerge from the data. These insights can help inform personalized marketing tactics aimed at engaging at-risk customers, such as targeted promotions or tailored content.
Moreover, customer feedback and satisfaction surveys play a crucial role in the retention process. Gathering feedback helps businesses understand customers’ pain points and preferences, allowing them to make informed adjustments to their offerings and improve overall satisfaction.
Case Studies: Success Stories of AI in Action
Real-world examples of companies successfully using AI for churn analysis illustrate the effectiveness of these strategies. For instance, some organizations have implemented AI tools that significantly reduced their churn rates by predicting customer behavior accurately and enabling proactive engagement.
By analyzing the specific strategies these companies employed, others can glean insights and adapt similar methods for their own operations. The key takeaways often revolve around the importance of data utilization, customer engagement, and continuous improvement in service delivery.
Conclusion
AI predictive customer churn analysis is no longer just a luxury—it’s a necessity for businesses aiming to thrive in 2025! By leveraging the power of artificial intelligence, organizations can not only predict churn but also take proactive steps to retain customers and enhance their overall experience. Start exploring AI tools today, implement effective retention strategies, and watch your customer loyalty soar! Let’s take your customer relationship management to the next level!