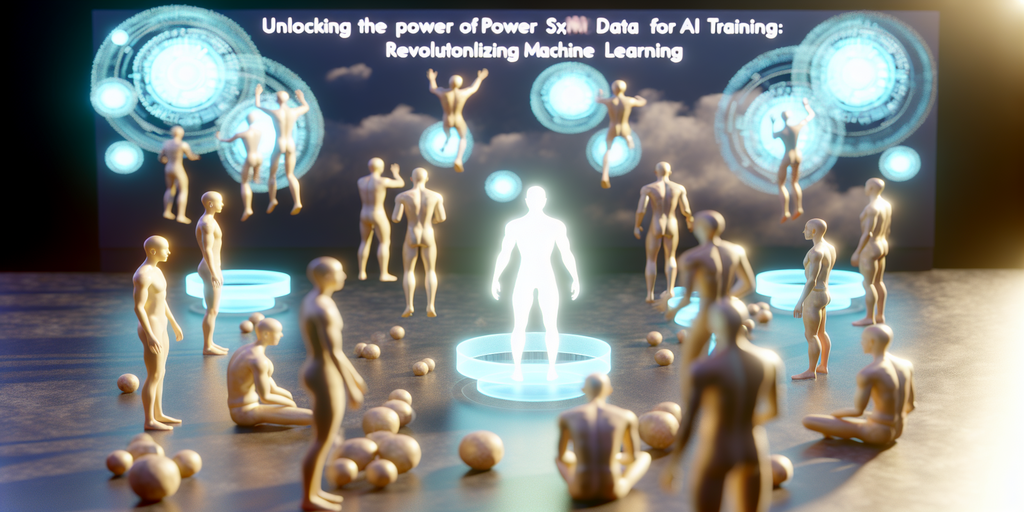
Unlocking the Power of Synthetic Data for AI Training: Revolutionizing Machine Learning in 2025
Discover how synthetic data for AI training is transforming machine learning. This comprehensive guide explores its benefits, challenges, and best practices for effective implementation.
Introduction
Did you know that using synthetic data for AI training can accelerate model development by up to 30%? In a world where data is the new oil, the demand for high-quality, relevant datasets is skyrocketing. However, acquiring and maintaining real data can often be costly and fraught with privacy concerns. This is where synthetic data steps in! By mimicking real-world data without the associated risks, synthetic data is paving the way for more efficient and ethical AI training practices. Join me as we delve into the fascinating realm of synthetic data, exploring its applications, benefits, and how to effectively integrate it into your AI training processes.
What is Synthetic Data?
Synthetic data refers to information thatâs artificially generated rather than obtained from real-world events. This type of data is created using algorithms and models that simulate the properties and characteristics of real data while avoiding privacy concerns. A key feature of synthetic data is its ability to mimic the statistical properties of actual datasets, making it useful for various applications, especially in fields like artificial intelligence (AI) and machine learning.
One major difference between synthetic data and real data is that synthetic data does not contain any personally identifiable information (PII), making it inherently safer for use in training AI models. Traditional datasets, on the other hand, may include sensitive information that requires strict privacy safeguards. Synthetic datasets can be customized to suit specific needs, providing flexibility that real-world data cannot always offer.
Some common sources of synthetic data include simulation software, generative models like Generative Adversarial Networks (GANs), and data augmentation techniques. These sources can create vast amounts of data that closely resemble real-world data without the associated risks.
The Benefits of Synthetic Data for AI Training
One of the most significant advantages of synthetic data is the ability to increase data volume without privacy issues. As organizations strive to comply with data protection laws, synthetic datasets provide a viable alternative for training models without risking sensitive information. This can lead to a larger amount of training data, enhancing the overall performance of AI systems.
Furthermore, synthetic data allows for the creation of diverse datasets. Diverse training data is crucial for improving model performance, as it helps ensure that AI systems can generalize well to new, unseen data. By generating various scenarios and conditions, synthetic data can improve the robustness of AI models.
Cost-effectiveness is another important benefit. The process of generating and labeling synthetic data can be significantly less expensive than collecting and preparing real data. This efficiency can lead to a more streamlined data acquisition process, saving both time and resources.
Additionally, synthetic data can accelerate the training process. With rapid data provisioning, developers can quickly obtain the datasets they need, enabling faster iterations and improvements in AI models.
Use Cases of Synthetic Data in AI Training
Synthetic data finds applications across a range of industries. In healthcare, for example, it can be used to create patient datasets for training diagnostic algorithms without compromising patient privacy. In finance, synthetic data can help in developing algorithms for fraud detection by simulating various transaction scenarios.
The automotive industry also benefits from synthetic data, particularly in the realm of autonomous vehicles. By generating realistic driving scenarios, companies can enhance the training of self-driving systems, preparing them for a multitude of real-world conditions.
Beyond these sectors, synthetic data plays a significant role in enhancing computer vision and natural language processing models. By providing diverse and plentiful training examples, it helps in addressing data scarcity, especially in specialized fields where real-world data may be limited.
Challenges and Considerations
Despite its benefits, the use of synthetic data comes with challenges that must be addressed. Quality control is paramount; synthetic datasets must be rigorously validated to ensure they accurately represent the scenarios they are meant to simulate. Poor-quality datasets can lead to misleading results and ineffective AI models.
Another concern is the potential for biases in generated data. Synthetic data is only as good as the algorithms that create it, and if these algorithms are trained on biased datasets, the synthetic data may inherit those biases. To mitigate this, itâs essential to implement strategies that promote fairness and reduce bias during the data generation process.
Legal and ethical considerations also come into play when using synthetic data. Organizations must navigate regulations and guidelines to ensure that their synthetic data practices align with legal standards. Transparency in data generation methods and use is vital for maintaining trust with stakeholders.
Best Practices for Implementing Synthetic Data
To effectively implement synthetic data, organizations should follow a series of best practices. First, itâs essential to establish clear goals for what you want to achieve with synthetic datasets. This includes understanding the specific needs of your AI models and the types of scenarios you want to simulate.
Next, employing the right tools and frameworks for synthetic data generation is crucial. There are various software solutions available that can help streamline the synthetic data creation process, making it easier to produce high-quality datasets.
Lastly, integrating synthetic data into existing training workflows requires careful planning. Organizations should ensure that synthetic datasets complement real data rather than replace it entirely. By combining both types of data, AI models can benefit from the strengths of each, leading to improved performance and accuracy.
Conclusion
In summary, synthetic data for AI training offers a myriad of advantages, from enhancing model accuracy to alleviating privacy concerns. While challenges exist, adopting best practices can lead you to harness its full potential. As we continue to advance in AI technologies, staying ahead of the curve with synthetic data will be crucial. Are you ready to revolutionize your AI training processes? Letâs explore the limitless possibilities of synthetic data together!